Experimental design is a crucial aspect of media studies research, as it allows researchers to test hypotheses about media effects and gain insights into the ways that media affects individuals and society. In this blog post, we will delve into the basics of experimental design in media studies and provide examples of its application.
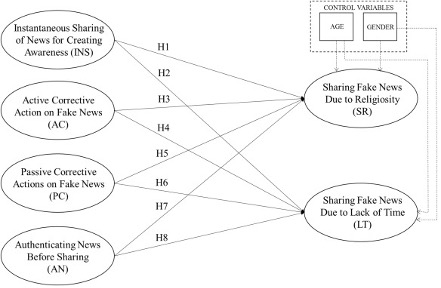
Step 1: Define the Research Question The first step in any experimental design is to formulate a research question. In media studies, research questions might involve the effects of media content on attitudes, behaviors, or emotions. For example, “Does exposure to violent media increase aggressive behavior in adolescents?”
Step 2: Develop a Hypothesis Once the research question has been defined, the next step is to develop a hypothesis. In media studies, hypotheses may predict the relationship between media exposure and a particular outcome. For example, “Adolescents who are exposed to violent media will exhibit higher levels of aggressive behavior compared to those who are not exposed.”
Step 3: Choose the Experimental Design There are several experimental designs to choose from in media studies, including laboratory experiments, field experiments, and natural experiments. The choice of experimental design depends on the research question and the type of data being collected. For example, a laboratory experiment might be used to test the effects of violent media on aggressive behavior, while a field experiment might be used to study the impact of media literacy programs on critical media consumption.
Step 4: Determine the Sample Size The sample size is the number of participants or subjects in the study. In media studies, sample size should be large enough to produce statistically significant results, but small enough to be manageable and cost-effective. For example, a study on the effects of violent media might include 100 adolescent participants.
Step 5: Control for Confounding Variables Confounding variables are factors that may affect the outcome of the experiment and lead to incorrect conclusions. In media studies, confounding variables might include individual differences in personality, preexisting attitudes, or exposure to other sources of violence. It is essential to control for these variables by holding them constant or randomly assigning them to different groups.
Step 6: Collect and Analyze Data The next step is to collect data and analyze it to test the hypothesis. In media studies, data might include measures of media exposure, attitudes, behaviors, or emotions. The data should be collected in a systematic and reliable manner and analyzed using statistical methods.
Step 7: Draw Conclusions Based on the results of the experiment, conclusions can be drawn about the research question. The conclusions should be based on the data collected and should be reported in a clear and concise manner. For example, if the results of a study on the effects of violent media support the hypothesis, the conclusion might be that “Exposure to violent media does increase aggressive behavior in adolescents.”
In conclusion, experimental design is a critical aspect of media studies research and is used to test hypotheses about media effects and gain insights into the ways that media affects individuals and society. By following the seven steps outlined in this blog post, media studies researchers can increase the reliability and validity of their results and contribute to our understanding of the impact of media on society.